종종 사전 학습된 딥러닝 모델을 불러와서 사용할 때 ckpt, pb, pth, pbtxt 등 다양한 확장자명을 볼 수 있는데 이중 .pb 확장자를 갖는 모델에 대한 글이다.
.pb 파일이란?
pb는 protocol buffer를 줄인 말로 protobuf라고도 부른다. 깊게 알 필요없이 pb는 데이터를 serialize하는 방식 중 하나인데 간단히 binary로 바꿔서 통신에 유리하도록 하는 방법이라고 보면 되겠다. 하나 기억해야 될 것은 serialize한 데이터의 구조도 같이 저장한다는 것이다.
위키피디아의 말을 빌리면 다음과 같이 설명하고 있다.
Protocol Buffers (Protobuf) is a method of serializing structured data. It is useful in developing programs to communicate with each other over a wire or for storing data. The method involves an interface description language that describes the structure of some data and a program that generates source code from that description for generating or parsing a stream of bytes that represents the structured data.
.pb로 저장된 딥러닝 모델
pb 파일에 대한 이해를 어느 정도 하고 난 뒤 다시 .pb 파일로 저장된 딥러닝 모델을 이해해보면, 사전학습된 모델 weight를 binary로 변환해서 저장한 형태라고 할 수 있으며, 동시에 일반 ckpt 파일과 달리 모델 구조에 대한 정보도 같이 저장하고 있다.
ckpt 모델만 갖고 있을 때는 모델 구조에 대한 정보를 따로 구해야 하고 테스트를 해보려고 해도 모델 구조에 대한 코드를 직접 작성해야 하는 고충이 있는데 pb 모델의 경우, 파일 자체가 모델 구조를 포함해서 binary화된 것이기 때문에 pb 파일만 갖고도 테스트가 가능한 장점이 있다.
.pb 파일에서 역으로 모델 구조 알아내기?
사용에서는 pb 파일이 아주 유용하지만 만약 구조와 weight를 알고 싶을 때는 역으로 더 어렵다. 마치 암호화가 더 된 버전에서 찾아내는 것과 같다. 대표적으로 google 에서 공개한 코드들은 웬만하면 .pb 형태로 inference만 가능한 형태로 많이 공유되기 때문에 .pb 파일로부터 구체적인 구조를 알아내려고 시도하게 되는 일이 잦은데 이 때마다 너무 번거롭다.
지금까지 내가 찾아낸 방식은 tensorboard를 이용해 .pb 파일 내 모델의 구조를 시각화하고, 시각화된 노드를 보면서 모델의 구조를 역계산하는 방법이다. 엄청나게 무식하고 쉽지 않은 일이긴 하지만 코드가 없는 상태에서 논문만 보고 찾는 것보다는 효과적이다.
.pb 파일 tensorboard로 시각화하기 (Tensorflow 1.X)
GitHub - tensorflow/tensorflow: An Open Source Machine Learning Framework for Everyone
An Open Source Machine Learning Framework for Everyone - GitHub - tensorflow/tensorflow: An Open Source Machine Learning Framework for Everyone
github.com
tensorflow github에서 예제로 제공해주는 코드가 있다. 저장된 모델이 있는 경로와 tensorboard 파일을 저장할 경로, 그리고 저장된 모델에 사용된 tag-set을 입력하면 바로 시각화해주는 코드다.
만약 tensorflow 1.X 처럼 버전 1을 쓸 경우, 위 코드가 그냥 동작할 것이다. tag-set은 모를 수 있는데 기본적으로 "serve", ",", "/", " " 중 하나를 넣어가면서 돌려보면 웬만하면 찾을 수 있을 것이다.
Tips
- 저장된 모델의 이름을 saved_model.pb로 변경해두고 위 코드를 사용해야 오류가 안 난다. 내부 코드에서 이름은 무조건 saved_model.pb라고 가정하고 돌아가더라.
.pb 파일 tensorboard로 시각화하기 (Tensorflow 2.X)
문제는 요즘 누가 tensorflow 버전 1을 쓰냐는 것인데 버전 2에서 위 코드를 쓸 경우, 절대 실행이 안된다. 정확히는 실행은 되고 코드도 정상적으로 끝나는데 막상 tensorboard를 켜보면 다음과 같은 오류가 뜨면서 시각화가 되지 않는다.
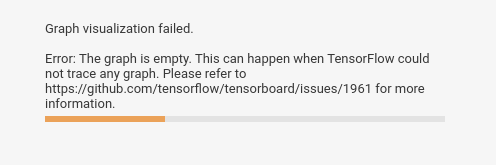
"Graph visualization failed" 문구와 함께 graph가 비어있다는 에러가 뜬다. 사전 학습된 모델이니 그래프가 비어있을 리 없는데 에러가 뜨는 것이므로 코드가 비정상적으로 동작했다는 것을 알 수 있다.
약간의 삽질 끝에 방법을 찾아냈는데 다음과 같이 수정하면 된다.
def import_to_tensorboard(model_dir, log_dir, tag_set):
"""View an SavedModel as a graph in Tensorboard.
Args:
model_dir: The directory containing the SavedModel to import.
log_dir: The location for the Tensorboard log to begin visualization from.
tag_set: Group of tag(s) of the MetaGraphDef to load, in string format,
separated by ','. For tag-set contains multiple tags, all tags must be
passed in.
Usage: Call this function with your SavedModel location and desired log
directory. Launch Tensorboard by pointing it to the log directory. View your
imported SavedModel as a graph.
"""
with session.Session(graph=ops.Graph()) as sess:
# input_graph_def = saved_model_utils.get_meta_graph_def(model_dir,
# tag_set).graph_def
###
model_path = os.path.join(model_dir, "saved_model.pb")
with tf.io.gfile.GFile(model_path, "rb") as f:
input_graph_def = tf.compat.v1.GraphDef()
loaded = input_graph_def.ParseFromString(f.read())
###
importer.import_graph_def(input_graph_def)
pb_visual_writer = summary.FileWriter(log_dir)
pb_visual_writer.add_graph(sess.graph)
print("Model Imported. Visualize by running: "
"tensorboard --logdir={}".format(log_dir))
graph를 불러오는 함수를 위와 같이 변경해주기만 하면 된다. 그러면 tensorboard 상에서 정상적으로 시각화되는 것을 볼 수 있다.

이제 이 시각화된 결과를 보고 모델을 역추적하는 고생을 하면 된다... 화이팅!
'Knowhow > Vision' 카테고리의 다른 글
Axis angle(Rodrigues notation) to Rotation matrix (0) | 2023.03.15 |
---|---|
Disparity generation (1) | 2023.03.08 |
Basalt 사용, tag 규격 정하기, 이미지로부터 rosbag 파일 만들기 (0) | 2023.01.19 |
Theseus tutorial : simple pose estimation (0) | 2022.11.24 |
COLMAP[CLI] SfM/MVS with known camera parameters and poses (0) | 2022.07.14 |